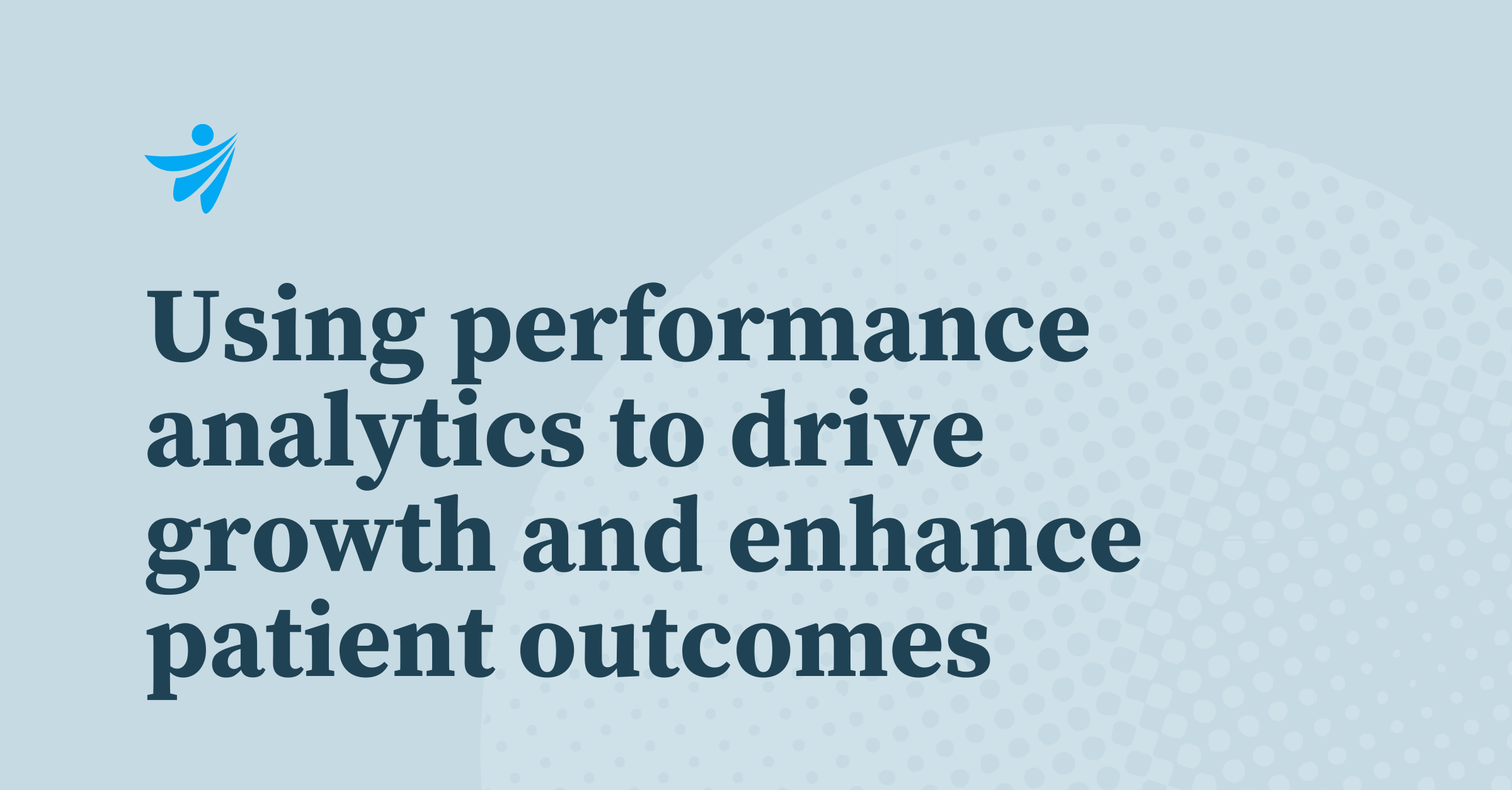
Insights for Providers
Jul 19, 2024
Insights for Providers | October 2, 2019
What will it take to shift the American health system from “sick care” to health care? Currently, health services primarily focus on disease treatment in the acute care setting rather than prevention. Primary care remains strapped with too few physicians who have too little time. Many Americans live in primary care health professional shortage areas, putting additional strain on the 61% of primary care physicians reporting symptoms of burnout.
This structure is fundamentally ill-equipped to manage the increasing burden of chronic disease. The 28% of Americans with 3+ chronic conditions account for 67% of health spending. Non-infectious diseases caused by obesity and poor nutrition are responsible for $480B in direct healthcare costs. These conditions are largely preventable. But they often require support beyond the four walls of a hospital or clinic. Shifting from “sick care” to healthcare requires a comprehensive understanding of Americans’ social context, actionable health analytics, and targeted care interventions to modify behaviors before they lead to chronic disease.
Current efforts to understand SDoH in healthcare typically utilize social determinant screenings or zip code level data. Surveys such as The Protocol for Responding to and Assessing Patients’ Assets, Risks, and Experiences (PRAPARE) are growing in popularity, but lack the scale to address the majority of patients. Under the CMS’s Accountable Health Communities model, only 24% of hospitals and 16% of physician practices have a system to screen for all five social needs (interpersonal violence, transportation, food insecurity, housing, and utility needs). And only a small proportion of their patients are likely screened. Due to limited time and competing priorities, physicians generally prioritize the sickest patients for risk assessments—once again focusing on “sick care,” not prevention.
Zip code and block-level data are being incorporated into data-savvy organizations, but there are limits on its ability to assess individual patient needs. Zip codes can exceed 100K Americans, but block groups are designed to range from 600–3K individuals. This limited methodology operates blindly to the sensitivity and tact needed to risk-stratify patients and address top social determinants of health. As a result, current efforts may stereotype patient journeys rather than providing the precise insights needed for individualized and personalized care.
At Clarify, we’ve actualized a different approach leveraging social determinants of health data and health analytics. With access to information from credit risk agencies, consumer databases, and public and private records that provide 400+ attributes for each individual American—we asked ourselves, is this information reliable and high quality for use inpatient care? And can the data used by big tech and major retailers for personalized marketing be used to better manage Americans’ health?
The answer is yes. But it takes work. Our team of epidemiologists, doctors, and data scientists developed a scalable methodology for assessing data quality—the Clarify Data Quality Index. It quantitatively evaluates social determinants of health data sets across seven dimensions, including missingness, information lag, and predictive power, among others. We broke ground in SDoH data validation, comparing consumer-sourced data against Census and aggregate statistics, as well as within and across our individually attributed data sources. The result was a dataset, available for >80% of Americans, with 400+ social determinants of health (SDoH) attributes spanning all 5 social needs—and ongoing additions as our list of validated attributes grow. This asset enables us to understand individuals’ social risk factors at scale, without relying on resource and time-intensive screenings. Additionally, it captures individuals who avoid interacting with the healthcare system and monitors changing needs over time.
How can this health analytic approach promote the shift from “sick care” to actual “healthcare?” Traditional approaches to risk-stratify patients, using instruments such as the LACE index and historical claims utilization often identify patients after their most costly events. These methods fail to surface rising risk. Predicting risk requires precise insight into disease burden and how SDoH in healthcare accelerates disease progression. Our health analytics prospective risk ratings incorporate clinical, utilization, and SDoH factors to drive a more holistic view of risk—for example, our palliative care model outperforms Elixhauser by 34%, identifies at-risk members an average of 5 months earlier than common clinical decision trees, and surfaces members with an average of 2.2x more utilization than industry models.
Bringing key information on individuals’ disease burden, care utilization, social context, and risk factors into one place—and translating that information into insights and action, dramatically impacts provider efficiency and intervention efficacy. Creating transparency into our risk ratings improves clinician buy-in and trust of the data, enabling providers to ask the right questions at the right time, making the most of their limited time with patients.