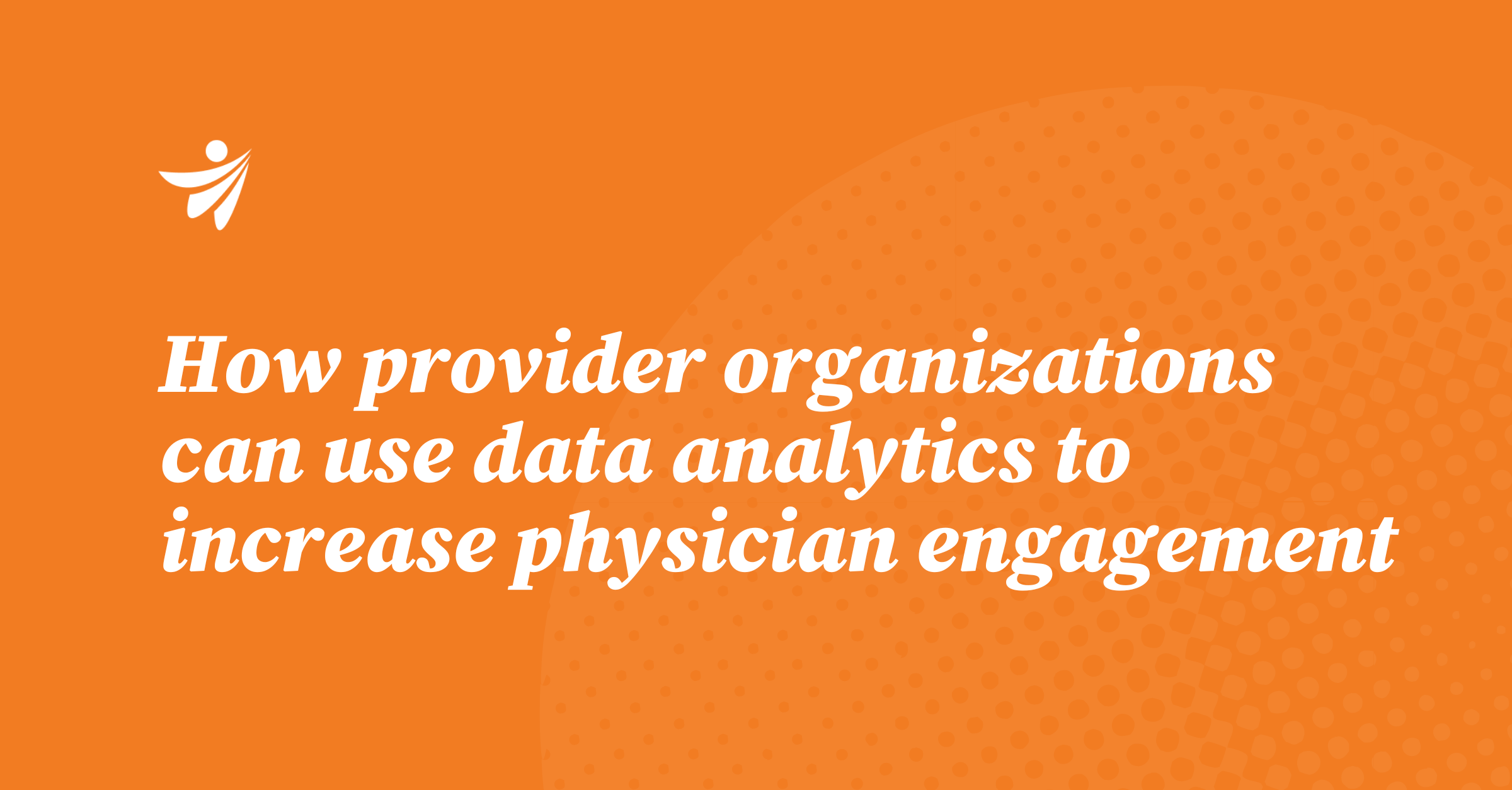
Insights for Providers
Apr 10, 2024
Healthcare Analytics | February 13, 2024
In healthcare, the ability to predict future demand with precision is not just a competitive advantage. For many hospitals and healthcare systems, it’s a necessity for survival. Optimizing resources to meet the moment calls for strategic planning powered by healthcare data analytics and effective demand forecasting. Demand forecasting in the context of hospital systems is critical for aligning resources like staff, equipment, and beds with future patient care needs. Healthcare providers need to make informed decisions while ensuring quality and maintaining operational efficiency. Growth teams play a critical part in this process, using healthcare data analytics to give providers the information they need to make the best decisions possible. However, the unpredictable nature of patient demand makes accurate forecasting a formidable task. Seasonal outbreaks and public health emergencies, for example, can present significant difficulties for even the best-prepared facilities. The complexity of operations in modern hospitals and healthcare systems only adds to the challenge. But with the following five strategies, growth teams can improve the accuracy of their forecasts. The result? A better chance of optimizing resources, improving patient outcomes, and enhancing the quality of care. Data analytics is the science of drawing insights from raw data. It is a broad term, encompassing a wide variety of objectives, as well as tools and processes, that vary from industry to industry. But any data analytics project begins with gathering data that are relevant to the goal, then pre-processing or “cleaning” the raw data, which often contains inaccuracies, structural differences, and duplications. In healthcare, demand forecasting begins with large datasets from sources like electronic health records (EHRs), billing platforms’ transaction logs, operational records, and patient portals, as well as external sources like social media, public databases, and economic indicators. For example, by examining patterns in a hospital’s admission rates and service utilization rates, growth teams can identify seasonal and cyclical trends that will likely be repeated. Growth teams often use sentiment analysis tools to identify these patterns and trends. Some sentiment analysis tools connect directly with applications like Zendesk and automatically tag support tickets. Other sentiment analysis tools sift through social media posts and other places a healthcare provider or brand is mentioned online. Both internal and external data can lead to insights on improving efficiency and reducing costs through better allocation of resources — and can help providers create better patient experiences and improve health outcomes. Data analysis can also provide the foundation for predictive modeling (discussed below) by identifying the key variables and relationships that have a bearing on the future. While data analysis is concerned with understanding what data shows about the past or present, predictive modeling uses that understanding (often derived from data analysis) to make informed predictions about unknown future events. Predictive modeling can employ statistical or machine learning models (or both), but data scientists always “train” these models with historical data (where the outcomes are known). Techniques can range from relatively simple linear regression to complex neural networks. For example, predictive modeling based on analysis of patient journeys from admission to follow-up can simulate patient flow. Insights about patient flow can both identify bottlenecks (and thus indicate ways to optimize that flow) and forecast demand for specific services or departments, improving the ability to scale care delivery and respond to patient needs. Predictive models also increase the accuracy of budgeting and financial forecasting. These projections can aid in things like optimizing inventory levels, identifying areas for cost savings and preventing overstaffing. Models can also accelerate time-to-market planning, as well as predict revenue, cash flow and expenses so healthcare leaders can adjust their strategies accordingly. External forces, including seasonal trends, public health issues, and local events, can all influence patient demand. For example, if the CDC expects influenza to be particularly transmissible in the upcoming winter season, health systems will likely need to plan for an uptick in hospitalizations. By incorporating such factors into predictive models, providers can better meet unexpected surges while maintaining quality standards. Data from social media platforms also provide insights into patients’ concerns and critiques. Public sentiment about a public health crisis could spike demand, while negative dialogue about an institution could damage its reputation and inhibit an organization’s growth. Anticipating any fluctuations in demand is critical to financial planning and resource management. Clinical, operational, and financial departments are often siloed, but collaboration between them can improve the accuracy of demand forecasting in healthcare. Sharing data (and insights) allows for integrated strategic planning and ultimately promotes better financial outcomes. The adoption of interoperability standards like Fast Healthcare Interoperability Resources (FHIR) is one way to enable better data across departments. FHIR helps to ensure that different healthcare IT systems can communicate effectively, share data, and interpret shared data correctly. Data sharing can also help providers adopt a holistic approach to patient care planning, particularly in optimized resource allocation at every point of patients’ care journeys. As economic conditions, consumer behavior, technology and market dynamics evolve, it’s critical to update predictive models with new data. This helps ensure that providers can adapt to the latest patient needs while improving care quality and accessibility. Refining forecasts also has financial benefits, including sustained operational efficiencies and cost savings. Over time, growth teams can analyze feedback and outcomes from previous forecasts to identify areas in which models may have been inaccurate. This feedback loop allows for adjustments and refinements that improve the models’ overall reliability. The frequency and methodology of updates can vary depending on several factors, including the model’s purpose, the availability of new data, and the model’s performance. Routine updates might occur quarterly or biannually, which typically allows the incorporation of recent data while balancing the resources required for updates. In some cases, specific events (such as the outbreak of a new disease, changes in healthcare regulations, or the introduction of new treatments) necessitate more frequent updates. During the COVID-19 pandemic, health systems continuously updated their models to predict the spread of the virus, hospitalization rates, and resource needs while incorporating new data on infection rates, vaccination rates, and public health policies. Some advanced models might employ continuous learning approaches, which update themselves in real-time or near-real-time as new data becomes available. However, this approach requires robust infrastructure and algorithms to ensure models’ stability. The journey to accurate demand forecasting requires both art and science. It requires rigorous analysis of sophisticated models as well as the ability to interpret data in the context of the broader healthcare landscape. But forecasting demand in healthcare isn’t just a mandate for growth teams — it’s a strategic imperative with implications for operations at every level. It’s also vital to promote a culture that values data-driven decision-making. By embracing these practices and anticipating demand with accuracy, hospitals and health systems can enhance the quality of patient care and drive sustainable growth for years to come.5 strategies to improve healthcare demand forecasting
1. Leverage advanced data analytics
2. Adopt predictive modeling techniques
3. Incorporate external factors into forecasts
4. Collaborate across departments
5. Engage in continuous improvement processes
Data-driven decisions powered by demand forecasting