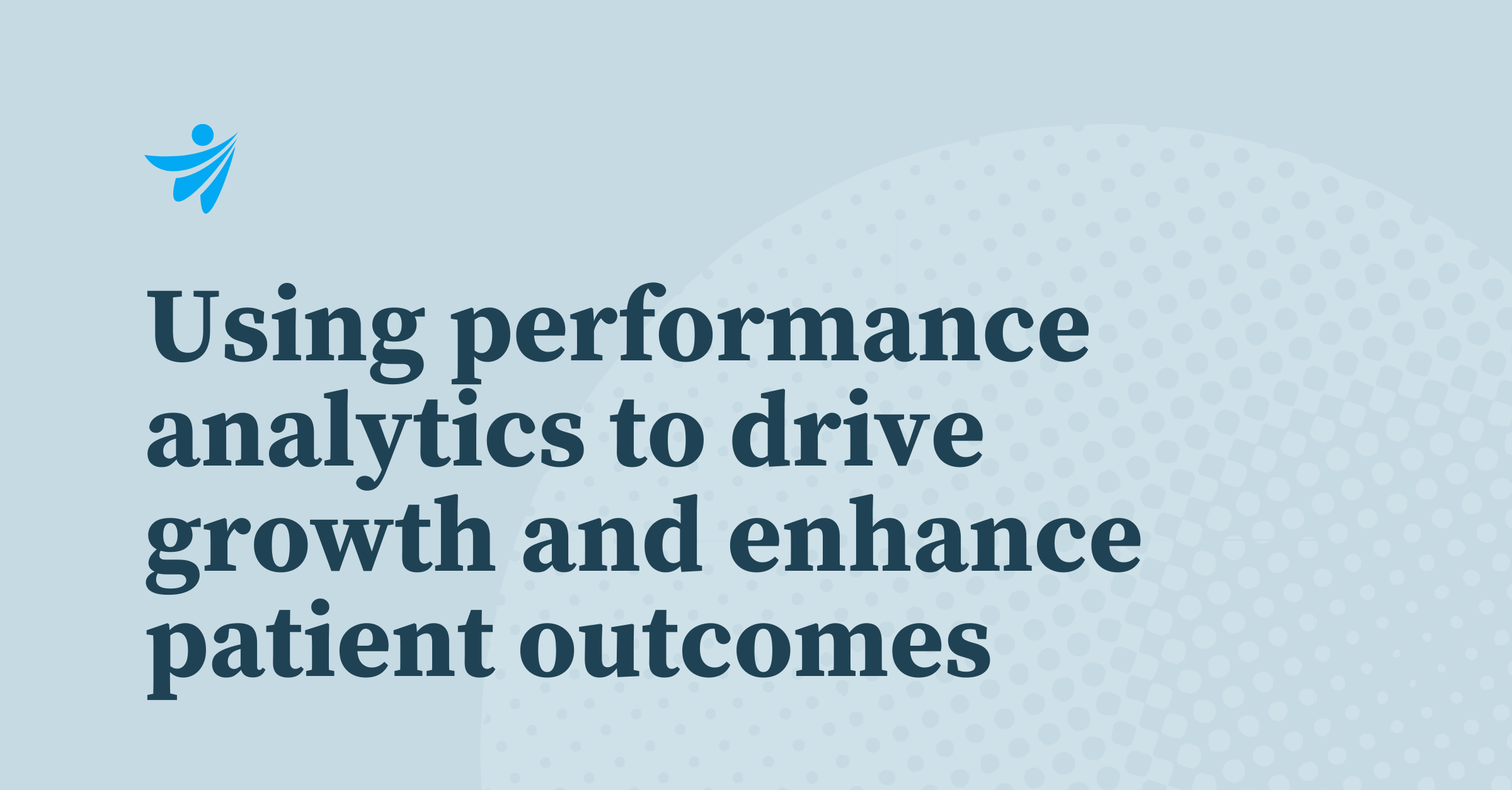
Insights for Providers
Jul 19, 2024
Healthcare Analytics | November 15, 2023
In the business of healthcare, time is of the essence. Health plans and care delivery organizations face steep competition for customers and patients, so it’s essential to move swiftly to gain customer loyalty and market share when launching a new health plan or service line. But, as the saying goes, only fools rush in. Healthcare leaders need to look at market trends, population health statistics, and other data to make informed growth decisions. This traditionally time-consuming work is critical to the success of any new venture. Predictive analytics allow for the best of both worlds: Models can parse through available data quickly, giving healthcare leaders the precise information they need to hit the market ahead of the competition while minimizing risk. Predictive analytics can help payers and providers streamline go-to-market planning and execution. With robust, high-quality datasets and the right predictive model, healthcare strategy leaders can gain a competitive edge to quickly and confidently launch products and services that will enhance care quality, control costs, and contribute to their future success. Innovative payers continually seek new ways to improve member experience, acquire and retain new customers, and manage risk. There are multiple ways health plan leaders can leverage accurate and dynamic predictive modeling to minimize the time it takes to get new offerings into the market. Launching new provider networks The traditional process of launching a new provider network is challenging because many plans lack reliable, accessible, and timely data on provider performance, market rates, and member disease burden — especially if the new network is in a new market. Using predictive analytics to accelerate go-to-market, a national health plan was able to design new high-performing Medicare Advantage networks in record time. They saved over 1,000 analyst hours and launched networks in 12 new markets in just three months — eight times faster than their typical timeline. Tapping into predictive modeling software that includes case-mix adjusted provider performance and other intelligence can help tell a story and surface insights for health plan leaders that would otherwise be buried or inaccessible. A shorter timeline in the planning and strategic phase means health plans can reap the rewards of lower costs, increased revenue, and improved member retention faster than ever before. Patients are increasingly acting as consumers with more options and autonomy when selecting where to receive care. That means it’s of utmost importance for provider organizations to deeply understand their patient population, allowing them to make informed growth decisions. Using predictive models to make strategic investment decisions will speed up the implementation of new programs, attract new patients, and gain their loyalty ahead of competitors. Three possible applications include: Expanding Service Lines Predictive analytics models can also help identify opportunities for service line expansion and speed the opening of those service lines. One large medical center wanted to expand its outpatient oncology service line but needed insight into its competitors and patient journeys to identify the best way forward. Leaders at the medical center used predictive analytics software to understand the patient, market, and service line intelligence they needed to take actionable steps to grow their market share. They uncovered a $50 million oncology opportunity and opened a new facility. Predictive analytics are an invaluable tool for healthcare leaders looking to gain a competitive edge and grow their organizations efficiently and effectively. They provide a strategic advantage by enabling faster, more informed decisions for market entry and service expansion, and empower both payers and providers to better identify risks, optimize pricing models, and predict customer behavior, thereby improving profitability and reducing time to market. Its real-world applications, from risk stratification to optimizing resource allocation, demonstrate its significant impact on enhancing care quality, controlling costs, and accelerating market responsiveness. Ultimately, predictive analytics is a key driver for healthcare organizations to move swiftly and intelligently, gaining market share and customer loyalty in an increasingly competitive landscape.Real-world applications: How predictive analytics accelerates time-to-market
Payers applications of predictive analytics
Provider applications of predictive analytics
Move swiftly and intelligently with predictive analytics