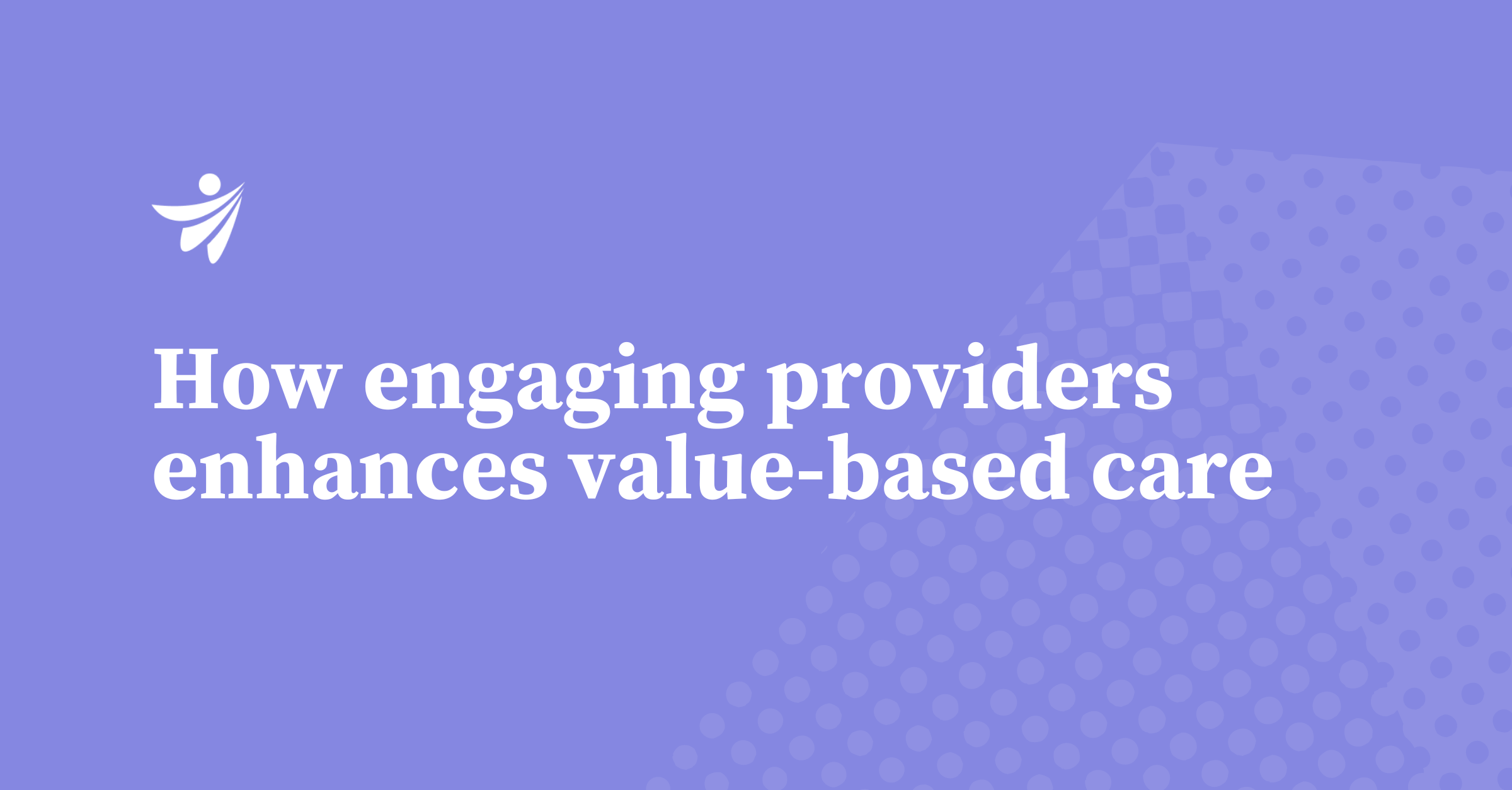
Healthcare Analytics
Jun 20, 2024
Healthcare Analytics | August 21, 2023
When it comes to healthcare data analytics, both quantity and quality play a critical role. While large and varied datasets (quantity) allow for big-data innovations like machine learning and AI, data quality is paramount to ensure our analyses lead us to fair, accurate, and transparent insights. Data quality is a measure of the condition of data based on factors such as accuracy, completeness, consistency, timeliness, and reliability is crucial to enabling both payers and providers to measure their performance and continually improve their policies, practices, and patient care. When it comes to matters of public and population health, we must hold ourselves to higher data quality standards. Population health management is best supported by a data-driven approach with a structured analytics process driven by skilled healthcare leaders and clinicians. According to the American Institute for Healthcare Management (AHIMA), the characteristics of quality data for use in population health are: Having access to more accurate data enables providers, payers, and policymakers to make better decisions for patient populations. However, many organizations struggle to assess, acquire, and maintain healthy datasets. Challenges such as fragmentation and inconsistency occur regularly, often because there are differences in how data is collected and managed. Many larger healthcare organizations are equipped with disparate systems storing volumes of data from multiple digital sources. So while they may have a lot of data, it’s not connected, potentially limiting its usefulness. One of the first steps in ensuring data quality in retrospective population health studies is implementing robust data cleaning and validation procedures, including data standardization and consistency checks. Data standardization results from mapping source data into a consistent format. Consistency checks identify missing and out-of-range values and other errors. Another best practice is to utilize data enrichment techniques for more comprehensive analysis. Some primary examples include incorporating demographic and sociodemographic data and augmenting clinical records with claims and billing data. Health plans, healthcare providers, and pharmaceutical companies leverage enriched claims analytics to enhance their operations, drive value, and improve outcomes for their patients. Some exciting applications of enriched claims data include: Identify critical junctures in a patient’s journey, evaluate care quality, assess referral patterns, and optimize resource allocation for providers. These enriched claims analyses lead to the identification of care gaps, assessment of treatment efficacy, and enhanced member satisfaction. Claims can also help organizations gain a deeper understanding of patient needs and preferences, enabling targeted interventions and more effective commercialization efforts. Evaluate the impact of virtual care on health outcomes, patient satisfaction, resource utilization, and cost-effectiveness. Through longitudinal analysis, stakeholders can uncover trends and associations over time, gaining insight into the benefits and limitations of virtual care. This analysis ensures fair comparisons between virtual care and traditional care models. Gain valuable insight into consumers’ healthcare service usage and preferences. This knowledge enables organizations to identify targeted marketing opportunities and enhance service offerings, ultimately increasing their share of healthcare spend. Enable predictive modeling and risk stratification for procedure outcomes. By developing predictive models to assess the likelihood of successful outcomes for specific procedures, stakeholders can identify patients at higher risk of complications or poor outcomes. This knowledge facilitates personalized interventions, pre-procedure optimization, and informed decision-making to improve overall patient care. Uncover utilization patterns, revealing areas of over-utilization or underutilization. These insights lead to informed decisions about resource allocation, improved access to care, and reduced waiting times for patients. With the right insights, organizations can enhance care coordination and management by identifying high-risk patients who require proactive interventions and personalized care plans. Empower analysts to evaluate the effectiveness of treatment programs and measure their impact on overall healthcare costs. By combining enriched claims with other sources like electronic health records and patient-reported outcomes, stakeholders can make evidence-based decisions, shape care guidelines, and optimize care management initiatives. Employing data governance and quality assurance measures is key for healthcare data quality. When the correct framework and structure are in place for a data governance program, healthcare organizations can realize a myriad of benefits, along with data quality, from standardized data processes, reduced operational costs, and improved security and compliance. By utilizing data governance and quality assurance measures, healthcare organizations can establish data quality metrics and benchmarks and conduct regular audits to produce data quality scorecards. When healthcare organizations receive vast amounts of data, it’s nearly impossible for all of it to be complete and error-free. That’s where a focus on data integration and interoperability comes in. Integration and interoperability are often used interchangeably but aren’t the same. Data integration means combining data from multiple disparate sources and connecting systems so data can be accessed by each one. Interoperability refers to the ability of those systems to communicate with each other and ensures they “speak the same language.” Both are necessary to provide high-quality retrospective data for population health management. Advancements in healthcare data analytics and machine learning algorithms also are making it easier for providers to ensure data quality. Artificial intelligence (AI) is being applied to data analysis on an increasing basis, resulting in the ability to automate and process data and extract insights from it that would typically require the expertise of a data scientist. Similarly, machine learning is being utilized to continuously monitor data, identify any errors, and notify of those anomalies. When healthcare organizations prioritize data quality, they can unlock many benefits, from direct patient care to scaling business operations to driving public health policy. Turning healthcare data into actionable insights presents a variety of challenges. Often, teams spend too much time trying to answer simple business questions, too much money processing data, and too much effort cleaning and ensuring quality. Thankfully, there are ways to get from data to insights faster, more cheaply, and with greater precision by partnering with the right vendor. Best-in-class data processing techniques utilize machine learning and other algorithms to create the best possible “single source of truth” to inform business decisions.The Role of Data Quality in Population Health
Best Practices for Ensuring Data Quality and Usability in Healthcare
Patient journey insights
Telehealth program impact
Share of wallet intelligence
Procedure performance and quality
Optimization of patient services
Enhancement of care management programs
Overcoming Data Quality Challenges in Healthcare
Applications of High-Quality Healthcare Data