The technology landscape is evolving at a pace that hasn’t been seen in years, and we’re at a generational stairstep in what is possible, not just in healthcare but everywhere. Generative artificial intelligence (AI) seems ubiquitous and has dramatic applicability in the healthcare industry. Organizations that have not already started integrating it into their systems need to prepare now. Just as this technology is disrupting other industries, generative AI can significantly increase the speed and efficiency of data interrogation to arrive at actionable conclusions in the healthcare industry. Companies need to lay down the framework now to understand what the disruption is going to look like and what it’s going to mean for healthcare. Clarify is already out in front. We just closed the beta launch of our generative artificial intelligence (AI) solution, Clara. This groundbreaking technology is set to empower healthcare delivery organizations to access insights into influenceable behavior changes that matter the most in lowering the cost and improving the quality of healthcare – at a fraction of the time it traditionally takes. Harnessing the power of Clarify’s healthcare claims dataset, one of the most comprehensive in the United States, Clara leverages advanced machine learning and natural language processing to quickly deliver precise, relevant, and actionable data to healthcare professionals. Clara’s closed beta launch signifies a significant step forward in Clarify Health’s commitment to transform healthcare through the power of data and AI. This capability offers the potential to transform healthcare delivery by enhancing the precision and speed of decision-making, leading to improved patient outcomes and reduced costs for patients and the healthcare system. Healthcare analysts and those who manage healthcare analysts must get ahead of where generative AI is going by asking how they can level up their capabilities with the help of these technologies. If you’re an analyst in any industry right now, you should be clamoring for access to these types of tools because they will become not state-of-the-art but state-of-the-norm. What do companies need in order to use data effectively for decision-making? It’s fairly straightforward. First, acquire data. Then refine that data, whether it’s simply just getting all the data into one accessible data lake or transforming the data into something more useful. Then you deploy a business intelligence tool on top of the data to start interrogating it. Then begins an iterative process of refining your queries to find the most useful data. This is the SQL model which has been in place for years and requires an analyst to really make sense of the data. Take the world of ChatGPT, for instance, isn’t an analyst really a chat interface on top of databases that supports iterative interrogation? Analysts need to rapidly interrogate data to solve their organization’s business questions. Generative AI transforms that data interrogation in minutes, saving analysts hours of work. There’s a massive locus of non-action in healthcare when it comes to adopting new technology; getting over that hump is hard. But with generative AI, you don’t have to retrain yourself or your team; you just simply have to ask questions as if you were talking to a colleague. Now, suddenly, generative AI gets much easier to adopt, and the conclusions get more focused and actionable. This breaks down one of the significant barriers to the adoption of technology that can drive better healthcare outcomes. Providers are trying to figure out which payers provide the best rates. A first-level generative interface on this is to simply ask, “What rate should I negotiate with an insurance company based on payments of competitors where I am of higher quality and I do significant volume.” You could arguably have done that with basic natural language processing tools that have been on the market for a while. But today’s tech stack makes this sort of work significantly easier and more intuitive. The hope is that analysts can turn days of drudgery into minutes of powerful responses. Analysts will evolve from being experts in tools like Tableau to becoming prompt engineers who know how to ask machines to give them the answers they need to do their jobs. That’s the leveling up we’re talking about. But that’s still all kindergarten relative to where we’re going. Again, sticking with our provider example, how can generative AI help providers invest their upstream influence to drive volume to their hospital? We talk about how we help payers enter a new market by going from spending two years developing a network and reducing that time down to months. Imagine if it took five minutes, and that’s what we’re talking about here. The ability to optimize a system using AI and design value-based care incentive structures is disruptive and probable within the next 12 months. We thought Siri was an advancement. Imagine an actual working interface where Siri can truly answer complex, multidimensional questions with a high degree of accuracy. The ability to solve incredibly complicated, fluid dynamic questions within minutes rather than days. For providers, this means identifying referral patterns so organizations can identify where to spend their marketing dollars to drive cases to their facilities. Rather than spending days building the interrogation of the data, providers can ask a prompt and receive an answer in minutes. And then even further… We are preparing for a reality in which these tools are being used to drive behavioral change to improve the value and reduce spending while retaining quality outcomes. How do you convince a doctor who is already delivering quality outcomes to change to do something different than what they’ve done? Putting it in the context of our payer partners, where they are today is that they spend a lot of time looking at underlying data sets to identify the small handful of items for each category of physician to make personalized next-best-action recommendations. For example, a patient presents for their annual wellness visit, and the next best action is a flu shot and a colonoscopy. But for this patient over here, the next best action is a COVID vaccine and breast cancer screening. That’s where we’re trying to go. How do you get a doctor to look at this information, to change behavior, and improve outcomes? Physician engagement rates for analytics offerings are low—less than 2%. Our current target is to get to 10%. We’re trying to get 1 in 10 doctors to look at a screen that shows them how they could perform better. But that’s the current state of where we all are in healthcare. We need to leapfrog the problem technologically. This means providing tools that are precise and actionable but also done in a way that’s much more consumable. That’s generative AI. Human validation will still be necessary. For the foreseeable future, there will need to be a human being that validates the answers coming back from generative AI. In the near term, which in healthcare might mean the next five years, you’re going to need somebody asking the question and then seeing the answer and then doing their traditional method of work to validate that generative AI’s answer is actually true. It’s faster, but it’s not as fast as it will eventually be. We must be really careful and cognizant of accuracy. We’re going to need to build a lot of validation architecture to be able to ask questions, see what the response is, and then go into the underlying dataset in these traditional ways of interrogating underlying data assets to validate that what is there is correct. And then the second significant caveat is bias. Bias in healthcare is a big issue because, in a grossly oversimplified way, generative AI is a pattern recognizer that makes recommendations. Saying simply that Black females with a high BMI get readmitted at a higher rate and just accepting it while not focusing on the socioeconomic context that causes that to happen builds bias into the system. If the historical patterns are inherently biased, then your recommendations are going to be biased too. How do we account for that? How do we adjust for that? It’s a big question that needs to be answered before generative AI can make real inroads in improving healthcare. We spent eight years and a lot of resources building what we believe is healthcare’s most curated patient and physician performance data set. If you think about that as a parallel to what Google did: they digitized as many books as they could get their hands on and then crowdsourced optical character recognition so that they had a massive English language and world language repository of how humans converse. Now, suddenly, they have a training data set to be able to answer the types of questions that are being answered right now. We collected and curated healthcare data for the purpose of deploying these same technologies that Google used on books for healthcare data. Generative AI is here now. We are actively working on folding it into our solutions with Clara’s help. Healthcare analysts need to be ready for what comes next.What is the future of AI in Healthcare?
Generative AI provides healthcare analysts with several use cases to significantly impact their organizations:
#1 Conversationally interrogating underlying data to negotiate rates.
#2 Multidimensional optimization:
#3 Conversational physician engagement:
Now, let’s call out some clear caveats to generative AI’s advancement.
Where does Clarify go from here?
- Author Details
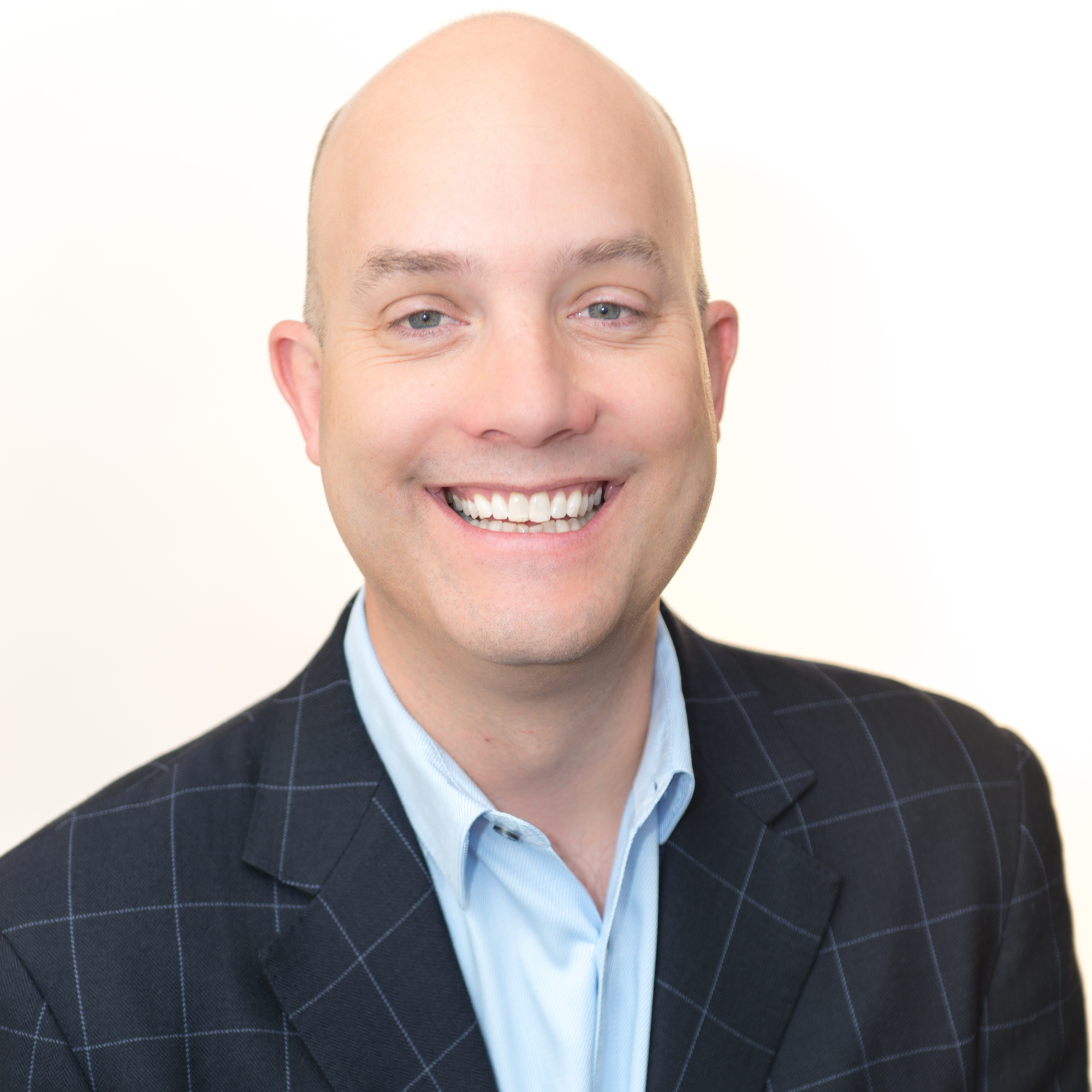